Recent Advancements in Krylov Methods and Their Applications
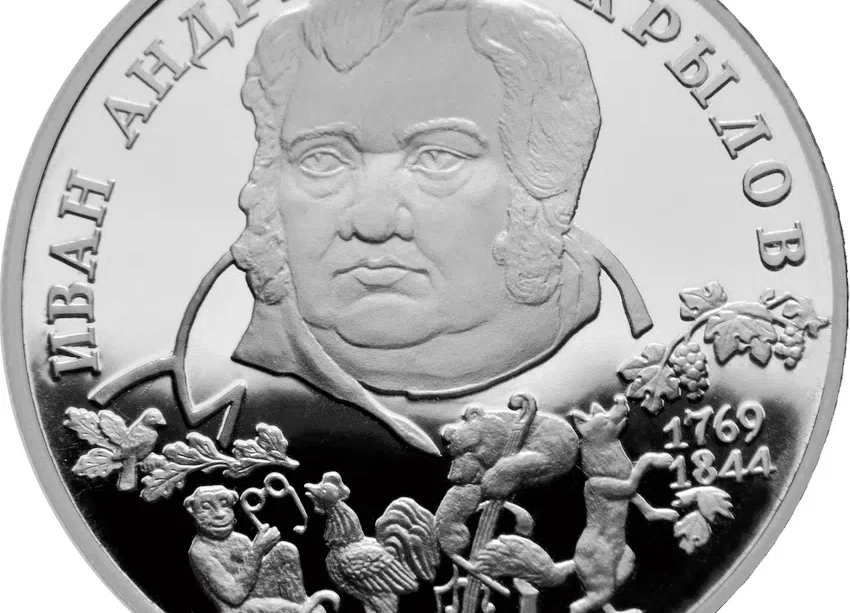
Introduction
The Krylov subspace methods have gained considerable traction in recent years, especially in fields such as artificial intelligence, computational mathematics, and robotics. Named after the Russian mathematician Aleksey Krylov, these methods are instrumental in solving large-scale linear systems and eigenvalue problems. Their increasing relevance is vital for scientists, engineers, and businesses aiming to leverage computational power in innovative ways.
Current Developments
In 2023, significant advancements in Krylov methods have been observed, particularly within AI research. These methods facilitate the acceleration of machine learning algorithms, enhancing their efficiency when processing vast datasets. For instance, researchers from the University of Toronto have published a paper detailing how Krylov subspace methods can improve convergence rates for deep learning models, thus reducing training times significantly.
In robotics, Krylov methods are being utilized in motion planning and control tasks. A recent project by a team at MIT used Krylov solvers to optimize the trajectory planning of robotic arms, allowing for smoother and more dynamic interactions with their environment. This has substantial implications for industries such as manufacturing and service robots, where precision and adaptability are key.
Facts and Figures
The ongoing research in Krylov methods has yielded promising results. According to a report by the Institute of Electrical and Electronics Engineers (IEEE), there has been a 35% increase in published research concerning Krylov applications in AI and robotics since 2020. Furthermore, businesses adopting this technology have reported gains in operational efficiency by up to 20%.
Moreover, collaborative efforts among various universities and tech firms aim to create standardized Krylov frameworks for easier implementation in future projects, fostering a more innovative and tech-savvy environment.
Conclusion
The future of Krylov methods seems bright, with growing applications across varied sectors. As technology continues to advance, the potential for these methods to revolutionize our approach to AI and robotics becomes clearer. With researchers and professionals dedicating substantial efforts to further refine Krylov techniques, we expect to see even more remarkable breakthroughs in the coming years. This is crucial for individuals and organizations looking to stay competitive in an ever-evolving tech landscape, providing them with the tools needed to harness complex computational problems.